Google DeepMind has made a significant advancement in their artificial intelligence (AI) research by presenting a new autoregressive model called “Mirasol3B” on Tuesday. The new model is aimed at improving the understanding of long video inputs and demonstrates a groundbreaking approach to multimodal learning, processing audio, video, and text data in a more integrated and efficient manner.
According to Isaac Noble, a software engineer at Google Research, and Anelia Angelova, a research scientist at Google DeepMind, who co-wrote a lengthy blog post about their research, the challenge of building multimodal models lies in the heterogeneity of the modalities. They explain that some of the modalities might be well synchronized in time (e.g., audio, video) but not aligned with text. Furthermore, the large volume of data in video and audio signals is much larger than that in text, so when combining them in multimodal models, video and audio often cannot be fully consumed and need to be disproportionately compressed. This problem is exacerbated for longer video inputs.
The new model, Mirasol3B, is an autoregressive multimodal model that subdivides learning into autoregressive modeling for time-aligned media modalities and non-time-aligned modalities. The model consists of an autoregressive component that fuses and jointly learns the time-aligned signals, which occur at different rates, and a non-autoregressive component that models the non-time-aligned modalities.
Google Research has been pushing towards multi-modal models that can flexibly handle many different modalities simultaneously, both as model inputs and as model outputs. The quality and capabilities of generative models for imagery, video, and audio have shown truly stunning and extraordinary advances in 2022. The new model, Mirasol3B, is a significant advancement in multimodal learning and processing audio, video, and text data in a more integrated and efficient manner.
A new approach to multimodal learning
Addressing the intricate nature of the task, Google’s Mirasol3B model strategically divides multimodal modeling by employing distinct autoregressive models. These models process inputs based on the specific characteristics of the modalities involved.
“Our approach involves incorporating an autoregressive element for modalities synchronized in time, such as audio and video, alongside a distinct autoregressive component for modalities that may not be temporally aligned but still follow a sequential pattern, such as text inputs like a title or description,” elucidate Noble and Angelova.
The announcement comes at a time when the tech industry is striving to harness the power of AI to analyze and understand vast amounts of data across different formats. Google’s Mirasol3B represents a significant step forward in this endeavor, opening up new possibilities for applications such as video question answering and long video quality assurance.
Google DeepMind has developed a new autoregressive model called “Mirasol3B” that aims to improve the understanding of long video inputs. The current models approach video modeling by extracting all the information at once, without sufficient temporal information. To address this, the new model applies an autoregressive modeling strategy where it conditions jointly learned video and audio representations for one time interval on feature representations from previous time intervals. This preserves temporal information.
The video is first partitioned into smaller video chunks, and each chunk can be 4-64 frames. The features corresponding to each chunk are then processed by a learning module called the Combiner, which generates a joint audio and video feature representation at the current step. This step extracts and compacts the most important information per chunk. Next, the joint feature representation is processed with an autoregressive Transformer, which applies attention to the previous feature representation and generates the joint feature representation for the next step. Consequently, the model learns how to represent not only each individual chunk but also how the chunks relate temporally.
The development of Mirasol3B marks a significant advancement in the field of multimodal machine learning. With its ability to handle diverse types of data and maintain temporal coherence in processing, the model yields state-of-the-art performance across several benchmarks while being more parameter-efficient than its predecessors.
Autoregressive models are powerful generative models that are well suited for data that appears in a sequence, modeling the probability of the current value, conditioned on previous ones. Video and audio information is sequential but also roughly time-synchronized. At the same time, other modalities, such as text, might be provided globally per video as context and applied to the full video rather than to specific parts. The autoregressive modeling of time-aligned video and audio is described in the Mirasol3B model. The model applies an autoregressive modeling strategy where it conditions video/audio representations corresponding to a time interval on feature representations from previous time intervals. These representations are learned jointly by the Combiner. The video is first partitioned into chunks, and the model processes each chunk autoregressively in time.
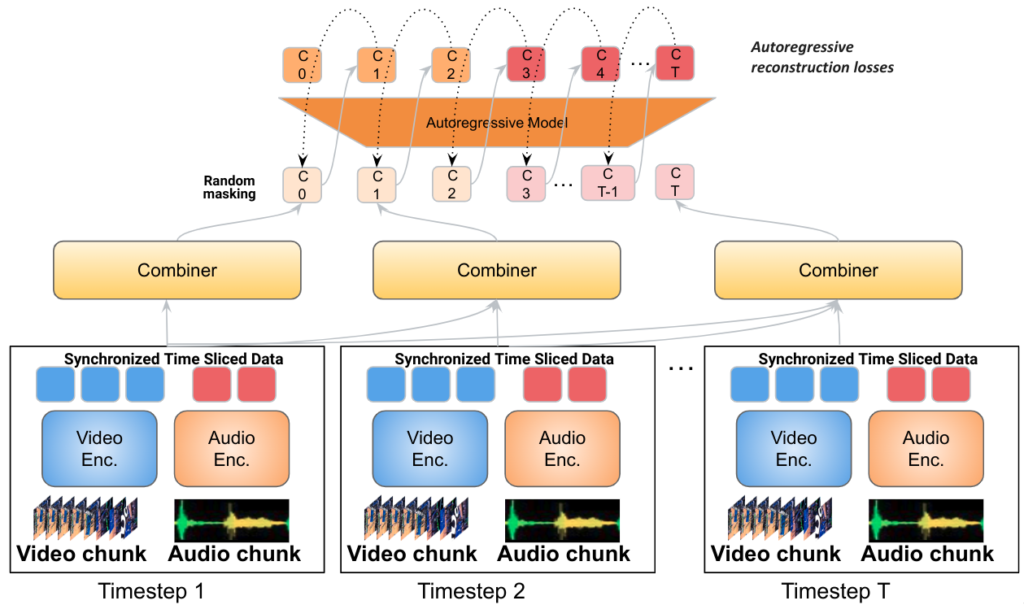
Potential applications for YouTube
Google may potentially explore using the Mirasol3B model on YouTube, the world’s largest online video platform and one of the company’s main sources of revenue. The model could be employed to enhance the user experience and engagement by providing more multimodal features and functionalities. These include generating captions and summaries for videos, answering questions and providing feedback, creating personalized recommendations and advertisements, and enabling users to create and edit their own videos using multimodal inputs and outputs.
For instance, the model could generate captions and summaries for videos based on both the visual and audio content, and allow users to search and filter videos by keywords, topics, or sentiments. This could improve the accessibility and discoverability of the videos, helping users find the content they are looking for more easily and quickly. Additionally, the model could be used to answer questions and provide feedback for users based on the video content, such as explaining the meaning of a term, providing additional information or resources, or suggesting related videos or playlists.
The potential applications of the Mirasol3B model on YouTube demonstrate the versatility and impact of multimodal machine learning in enhancing user experiences and interactions with video content. By leveraging the model’s capabilities, YouTube could further enrich its platform with advanced features that cater to the diverse needs and preferences of its users, ultimately contributing to a more engaging and personalized viewing experience.
A mixed reaction from the AI community
The announcement of the Mirasol3B model has sparked significant interest and excitement within the artificial intelligence community, while also eliciting some skepticism and criticism. Several experts have commended the model for its versatility and scalability, expressing optimism about its potential applications across various domains.
For example, Leo Tronchon, an ML research engineer at Hugging Face, expressed his interest in models like Mirasol that incorporate more modalities, particularly audio and video. He highlighted the scarcity of strong models in the open that utilize both audio and video, emphasizing the potential usefulness of such models on platforms like Hugging Face.
On the other hand, Gautam Sharda, a computer science student at the University of Iowa, raised concerns about the absence of code, model weights, training data, or an API for the Mirasol3B model. He expressed a desire to see the release of tangible assets beyond just a research paper.
The diverse reactions to the announcement of the Mirasol3B model reflect the complex landscape of AI research and development, encompassing both enthusiasm for technological advancements and critical evaluation of practical implementation and accessibility.
A significant milestone for the future of AI
The introduction of the Mirasol3B model represents a significant milestone in the field of artificial intelligence and machine learning, showcasing Google’s ambition and leadership in developing cutting-edge technologies that have the potential to enhance and transform human lives. However, this advancement also presents a challenge and an opportunity for researchers, developers, regulators, and users of AI. It is crucial to ensure that the model and its applications align with the ethical, social, and environmental values and standards of society.
As the world becomes more multimodal and interconnected, fostering a culture of collaboration, innovation, and responsibility among stakeholders and the public is essential. This will contribute to creating a more inclusive and diverse AI ecosystem that can benefit everyone.
The announcement has generated a mix of interest and skepticism within the artificial intelligence community. While some experts have praised the model for its versatility and scalability, others have raised concerns about the absence of tangible assets beyond the research paper, such as code, model weights, training data, or an API. This diversity of reactions reflects the complex landscape of AI research and development, encompassing both enthusiasm for technological advancements and critical evaluation of practical implementation and accessibility.
In conclusion, the introduction of the Mirasol3B model underscores the potential of multimodal machine learning to revolutionize various domains, while also highlighting the importance of ethical considerations and responsible deployment. It represents a significant step forward in advancing AI capabilities and underscores the need for collaborative efforts to ensure that these advancements are aligned with societal values and standards.
Sources:
https://venturebeat.com/ai/google-deepmind-breaks-new-ground-with-mirasol3b-for-advanced-video-analysis/
https://blog.research.google/2023/11/scaling-multimodal-understanding-to.html?m=1
http://blog.research.google/2023/01/google-research-2022-beyond-language.html?m=1
https://arxiv.org/pdf/2311.05698.pdf
https://www.linkedin.com/posts/googleresearch_introducing-mirasol-a-multimodal-model-for-activity-7130323647172362241-JP6h?trk=public_profile_like_view
https://openaccess.thecvf.com/content/CVPR2023/papers/Yoo_Towards_End-to-End_Generative_Modeling_of_Long_Videos_With_Memory-Efficient_Bidirectional_CVPR_2023_paper.pdf
https://openreview.net/forum?id=rJgsskrFwH
https://the-decoder.com/googles-mirasol-pushes-the-boundaries-of-ai-video-understanding/
https://miro.medium.com/v2/resize:fit:875/0*7zXqcdgXIgyqnwxy.png