
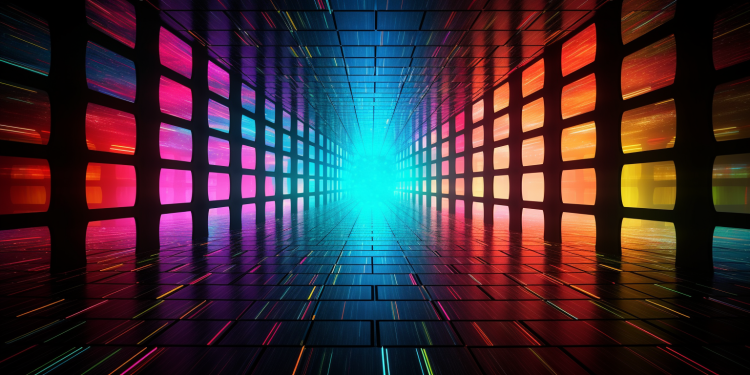
In the past year, there has been a significant surge in the influence of AI, prompting questions about whether it is on the brink of dominating humanity, merely a passing technological trend, or a more complex phenomenon. The situation is intricate. While ChatGPT’s achievement of passing the bar exam is undeniably impressive, it raises concerns, especially for lawyers. Nevertheless, there are emerging issues with the software’s capabilities, exemplified by an incident where a lawyer utilized ChatGPT in a courtroom, leading to the bot generating false elements in their arguments.
AI is poised to make further strides in its abilities, yet significant uncertainties persist. Ensuring our confidence in AI remains a challenge. We must guarantee that its results are not only accurate but also devoid of bias and censorship. Moreover, it’s essential to understand the origins of the data used to train AI models and ascertain that it wasn’t manipulated.
Tampering creates high-risk for all AI models, with even greater consequences for those earmarked for applications in safety, transportation, defense, and other domains where human lives are in jeopardy.
Even as national agencies worldwide recognize the growing significance of AI within our procedures and frameworks, this doesn’t imply that adoption should proceed without meticulous attention. The key inquiries that require resolution are as follows:
If we know that a model has been trained to its designed purpose, and we know exactly where it is being deployed (and what it can do), then we have eliminated a significant number of risks in AI being misused.
There are many different methods to verify AI, including hardware inspection, system inspection, sustained verification and Van Eck radiation analysis.
Hardware inspections are physical examinations of computing elements that serve to identify the presence of chips used for AI. System inspection mechanisms, by contrast, use software to analyze a model, determine what it’s able to control and flag any functions that should be off-limits.
This system operates by identifying and isolating a system’s quarantine zones, which are intentionally obscured to safeguard intellectual property and confidential information. Instead, the software examines the transparent components in the vicinity to identify and highlight any AI processing within the system, all while keeping sensitive data and intellectual property undisclosed.
Ongoing validation procedures take place subsequent to the initial inspection, serving to guarantee that once a model is put into operation, it remains unaltered and free from tampering. Certain anti-tampering methods, including cryptographic hashing and code obfuscation, are integrated directly within the model.
Cryptographic hashing enables an examiner to identify alterations in the foundational state of a system while safeguarding the confidentiality of the underlying data or code. Code obfuscation methods, still in early development, scramble the system code at the machine level so that it can’t be deciphered by outside forces.
Van Eck radiation analysis examines the radiation pattern emitted during a system’s operation. Given that intricate systems execute multiple parallel processes, the radiation emissions often appear scrambled, making it challenging to extract particular code. Nonetheless, the Van Eck method can identify significant alterations, such as the introduction of new AI, without the need to decipher any confidential information that the system’s operators aim to protect.
Most importantly, it’s crucial to ensure the authenticity of the data supplied to an AI model right from the source. To illustrate, consider a scenario where an adversary, rather than attempting to physically destroy your fleet of fighter jets, chooses to manipulate the training data used for instructing the signal processing AI model of these jets. Every AI model relies on data for its training, which shapes how the model comprehends, analyzes, and responds to new inputs. While the process of training involves a substantial amount of technical intricacies, it fundamentally revolves around aiding AI in grasping information in a manner akin to human understanding. The process is analogous, as are the potential pitfalls.
Ideally, our training dataset should mirror the actual data that the AI model will encounter post-training and deployment. For instance, one could compile a dataset of previous employees with exceptional performance records and employ those characteristics to train an AI model capable of evaluating a potential candidate’s qualifications based on their resume.
An example of this approach is Amazon’s experience. The outcome? From an objective standpoint, the model proved highly successful in performing its designated task. The downside? The data unwittingly instilled bias in the model. The majority of top-performing employees in the dataset happened to be male, leading to two possible conclusions: either men outperformed women, or the dataset was skewed due to a higher male hiring rate. The AI model lacks the capacity to consider the latter and, consequently, had to assume the former, giving undue weight to a candidate’s gender.
The establishment of verifiability and transparency is crucial for crafting AI that is secure, precise, and ethical. End-users deserve to be informed that the AI model was trained on the right data. Utilizing zero-knowledge cryptography to verify the data’s integrity offers assurance that AI is trained on unaltered, accurate datasets right from the outset.
Business leaders should possess a fundamental understanding of available verification methods and their effectiveness in identifying AI usage, model changes, and biases in the original training data. The initial step is to identify solutions. The platforms responsible for developing these tools act as a crucial defense against potential issues caused by dissatisfied employees, industrial or military espionage, or human errors, which can lead to significant problems with potent AI models.
While verification may not address every challenge in AI-based systems, it plays a significant role in ensuring that AI models function as intended and are promptly recognized if they undergo unexpected alterations or tampering. Given the increasing integration of AI into our daily lives, it’s imperative to establish trust in this technology.
Sources: