Recently, there have been many issues related to academic misconduct,...
Read More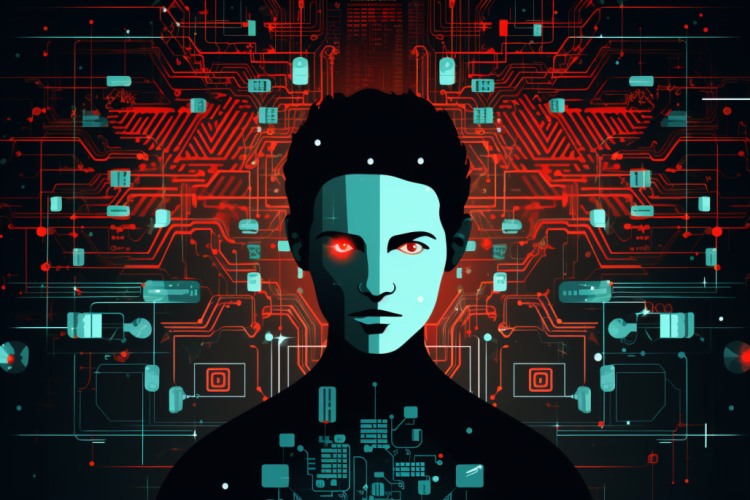
The rapid rise of large-scale language models (LLMs) and generative AI poses new challenges for security teams everywhere. Generative AI, which creates new ways to access data, does not fit into the traditional security paradigm that focuses on preventing data from going to people who should not have it.
To help organizations respond quickly to generative AI without introducing undue risk, security providers need to update their programs to take into account the new types of risks and how they will put pressure on existing programs.
Entire industries are currently being built and extended on top of LLMs hosted by services such as OpenAI, Hugging Face, and Anthropic. In addition, there are a number of open models available, such as Meta’s LLaMA and OpenAI’s GPT-2. Access to these models could help an organization’s employees solve business challenges. However, for a variety of reasons, not everyone is in a position to directly access these models. Instead, employees often look for tools that promise easy access to the models, such as browser extensions, SaaS productivity applications, Slack apps, and paid APIs.
These intermediaries are becoming a new source of shadow IT. Using Chrome extensions to write better sales emails feels less like using a vendor and more like a productivity hack. For many employees, it is not obvious that by sharing all of this with third parties, they are inviting the leakage of important sensitive data, even if the organization has no problem with the underlying model or the providers themselves.
This type of risk is relatively new to most organizations. Three potential boundaries are associated with this risk:
The problem in these cases is understanding what data will be used for the model. Only individuals with access to training data, i.e., data for fine-tuning, should have access to the resulting model. As an example, suppose an organization is using a product that uses the contents of a productivity suite to fine-tune LLMs. How would that tool ensure that I cannot use the model to retrieve information from a document to which I do not have access rights? Furthermore, how will it update its mechanisms after my original access rights are revoked? These are tractable issues, but they require special consideration.
While privacy considerations are nothing new, the use of AI to handle personal information can make these issues particularly challenging. In many jurisdictions, automated processing of personal information to analyze or predict certain aspects of a person is a regulated activity; the use of AI tools adds nuance to such processing and may make compliance with requirements such as providing opt-outs more difficult. Another consideration is how training and fine-tuning models for personal information will affect their ability to respond to deletion requests, restrictions on data reuse, data residency, and other challenging privacy and regulatory requirements.
Vendor security, enterprise security and product security are particularly stretched by the new types of risk introduced by gen AI. Each of these programs needs to adapt to manage risk effectively going forward. Here’s how.
The starting point for vendor security when deploying AI tools is to treat these tools like any other tools you deploy from other vendors. Make sure they meet the usual requirements for security and privacy. Your goal is to ensure that the vendor is a trusted custodian of your data. Given the newness of these tools, many vendors may be using them in less than the most responsible manner. Therefore, you need to add considerations to your due diligence process.
For example, we might consider adding questions to our standard questionnaire:
Your due diligence may take a different form, and I am sure that many standard compliance frameworks such as SOC 2 and ISO 27001 will incorporate relevant controls into future versions of the framework. And I am sure that many of the standard compliance frameworks, such as SOC 2 and ISO 27001, will incorporate the relevant controls into future versions of the framework.
Balancing friction and usability varies from one organization to another. Your organization might already have stringent controls regarding browser extensions and OAuth applications in your SaaS environment. Now is an excellent time to reevaluate your approach to ensure it strikes the right balance. Untrusted intermediary applications often take the form of easily installable browser extensions or OAuth applications connecting to existing SaaS applications. These are observable and controllable vectors.
The risk of employees using tools that transmit customer data to an unauthorized third party is especially potent now that many of these tools offer impressive solutions using generative AI. In addition to technical controls, it’s crucial to set expectations with your employees and assume good intentions. Ensure that your colleagues understand what is acceptable and what isn’t concerning the use of these tools. Collaborate with your legal and privacy teams to develop a formal AI policy for employees.
The most significant change in product security involves ensuring that you do not become an untrusted intermediary for your customers. Clearly explain in your product how you use customer data with generative AI. Transparency is the most powerful tool in building trust. Your product should also respect the same security boundaries that your customers expect. Do not allow individuals to access models trained on data they cannot directly access. In the future, there may be more mainstream technologies to apply fine-grained authorization policies to model access, but we are still in the early stages of this significant shift. Prompt engineering and prompt injection are intriguing new areas of offensive security, and you do not want your use of these models to become a source of security breaches. Give your customers options, allowing them to opt in or opt out of your generative AI features. Ultimately, it’s essential not to hinder progress. If these tools will make your company more successful, avoiding them due to fear, uncertainty, or doubt may be riskier than fully engaging in the conversation.
Source : https://venturebeat.com/security/generative-ai-a-pragmatic-blueprint-for-data-security/
Recently, there have been many issues related to academic misconduct,...
Read MorePrihardanu et al. (2021) analyzed survey data related to indoor...
Read MoreBigBear.ai is a company at the forefront of leveraging artificial...
Read More