Recently, there have been many issues related to academic misconduct,...
Read More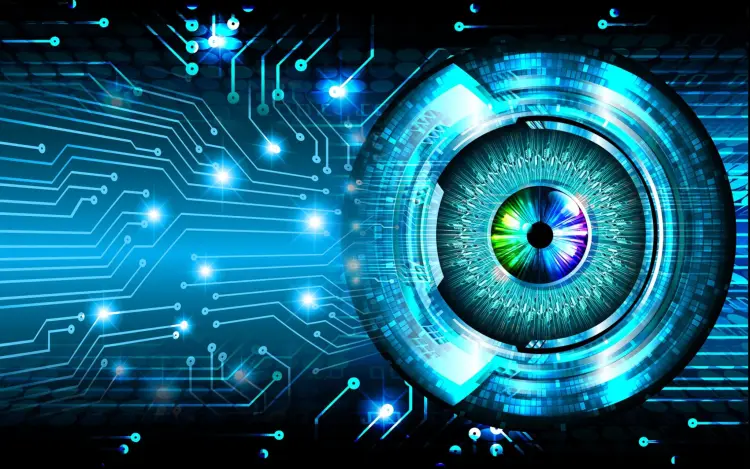
Computer Vision (CV) has seen rapid advancements in recent years, permeating various aspects of our daily lives. While it may appear as a novel and exciting innovation to the average person, the reality is quite different.
CV has been undergoing evolution for several decades, with foundational studies dating back to the 1970s laying the groundwork for many of the algorithms we utilize today. Approximately a decade ago, a nascent technique emerged, still in the theoretical stages: Deep learning, an AI approach that harnesses neural networks to tackle exceptionally intricate problems, provided you possess the requisite data and computational resources.
As deep learning continued to mature, its aptitude for resolving specific CV challenges became evident. Tasks like object detection and classification proved particularly amenable to the deep learning paradigm. Consequently, a division began to emerge between “traditional” CV, reliant on engineers’ mathematical and geometric problem-solving skills, and deep learning-based CV.
However, it’s essential to note that deep learning didn’t make traditional CV obsolete. Both branches continued to advance, illuminating which challenges are best suited for resolution through big data and which should still be tackled using mathematical and geometric algorithms.
The transformative potential of deep learning in the realm of computer vision hinges on the availability of suitable training data or the existence of discernible logical or geometric constraints that can guide the network in autonomously refining its learning.
Historically, classical computer vision was tasked with object detection, the identification of features such as edges, corners, and textures (known as feature extraction), and even the pixel-level labeling of images (referred to as semantic segmentation). However, these processes were arduous and demanding.
The detection of objects necessitated expertise in techniques like sliding windows, template matching, and exhaustive search. Extracting and categorizing features required engineers to devise customized methodologies. The intricate task of differentiating between various object classes at the pixel level entailed a substantial amount of effort to delineate distinct regions, and even experienced computer vision engineers did not always achieve accurate differentiation for every pixel in an image.
Specifically, convolutional neural networks (CNNs) and region-based CNNs (R-CNNs) have made this task considerably routine, particularly when coupled with extensive labeled image datasets from industry giants like Google and Amazon. With a well-trained network, there’s no need for explicit handcrafted rules, and these algorithms excel in detecting objects from various angles and under diverse conditions.
In the realm of feature extraction, deep learning offers a streamlined approach that relies on competent algorithms and diverse training data to prevent model overfitting and achieve high accuracy when dealing with new production data. CNNs, in particular, excel in this domain. Furthermore, when applied to semantic segmentation, the U-net architecture has demonstrated exceptional performance, eliminating the complexity of manual processes.
However, while deep learning has brought about a paradigm shift, it’s worth noting that for specific challenges addressed by simultaneous localization and mapping (SLAM) and structure from motion (SFM) algorithms, classical computer vision solutions still outperform newer approaches. These concepts involve utilizing images to comprehend and map the dimensions of physical spaces.
SLAM is primarily concerned with constructing and continually updating maps of an area while simultaneously tracking an agent (typically a robot) within that map. This technology is pivotal in enabling autonomous driving and the operation of robotic vacuum cleaners.
Similarly, SFM relies on advanced mathematical and geometric principles, aiming to create a 3D reconstruction of an object using multiple views obtained from an unordered set of images. It proves valuable when real-time responses are not necessary.
Initially, it was believed that substantial computational power would be essential for effective SLAM implementation. However, classical computer vision pioneers devised approaches that significantly reduced computational demands through approximations.
SFM, in contrast, is even more straightforward. Unlike SLAM, which often involves sensor fusion, SFM relies solely on the intrinsic properties of the camera and the image’s features. This cost-effective method offers a reliable and accurate representation of objects, especially when laser scanning is impractical due to limitations in range and resolution.
There remain challenges that deep learning cannot address as effectively as classical computer vision, and engineers should persist in employing traditional methods to tackle such issues. When intricate mathematics and direct observation are integral, and obtaining an appropriate training dataset is challenging, deep learning proves to be excessively potent and unwieldy to yield an elegant solution. An apt analogy here is that of a bull in a China shop: just as ChatGPT is not the most efficient (or accurate) tool for basic arithmetic, classical computer vision will continue to excel in specific challenges.
This gradual shift from classical to deep learning-based computer vision leaves us with two key insights. Firstly, we must recognize that complete replacement of the old with the new, while simpler, is not always the right approach. When a field experiences disruption from new technologies, it’s crucial to meticulously assess each case to determine which problems benefit from new techniques and which still find better solutions in older approaches.
Secondly, while this transition offers scalability, there’s a touch of nostalgia involved. Classical methods were more manual, but they also encompassed both art and science. Creativity and innovation in extracting features, identifying objects, discerning edges, and capturing essential elements were driven by profound thinking, rather than just deep learning.
As we move away from classical computer vision techniques, engineers like myself sometimes find themselves evolving into integrators of computer vision tools. While this shift is “good for the industry,” it’s somewhat wistful to leave behind the artistic and creative aspects of the role. A forthcoming challenge will be to find ways to incorporate this artistic dimension in different ways.
Over the next decade, I anticipate that “understanding” will gradually supplant “learning” as the primary focus in network development. The emphasis will shift from simply how much the network can learn to how deeply it can comprehend information, and how we can facilitate this comprehension without inundating it with excessive data. Our aim should be to empower the network to derive profound insights with minimal intervention.
The next ten years are bound to bring surprises in the field of computer vision. Perhaps classical computer vision will eventually become obsolete, or maybe deep learning will be surpassed by an as-yet-unseen technique. However, for the time being, these tools remain the best options for addressing specific tasks and will serve as the foundation for the continued evolution of computer vision in the coming decade. Regardless, it promises to be an exciting journey.
Recently, there have been many issues related to academic misconduct,...
Read MorePrihardanu et al. (2021) analyzed survey data related to indoor...
Read MoreBigBear.ai is a company at the forefront of leveraging artificial...
Read More